What is Projection?#
Author : Mustafa Sadeghi
Contact : mustafasadeghi@mail.um.ac.ir
Definition of Projection in #
The projection of a vector
In this formula:
represents the dot product of and , which measures how much aligns with . represents the magnitude (length) of vector , ensuring that the projection accounts for the size of .The result is a scaled version of
that points in the same direction as , but has a magnitude such that the difference between and its projection is minimized.
In summary, projection is the transformation that gives the closest vector along the direction of
import numpy as np
import matplotlib.pyplot as plt
import math
# line b
b = np.array([ 4,1 ])
# line a
a = np.array([ 2, 5 ])
# beta
beta = (a.T@b) / (a.T@a)
# draw!
plt.plot([0, b[0]],[0, b[1]],'r',label='b')
plt.plot([0, a[0]],[0, a[1]],'b',label='a')
# now plot projection line
plt.plot([b[0], beta*a[0]],[b[1], beta*a[1]],'--',label=r'b-$\beta$a')
plt.axis('square')
plt.grid()
plt.legend()
plt.axis((-6, 6, -6, 6))
plt.show()
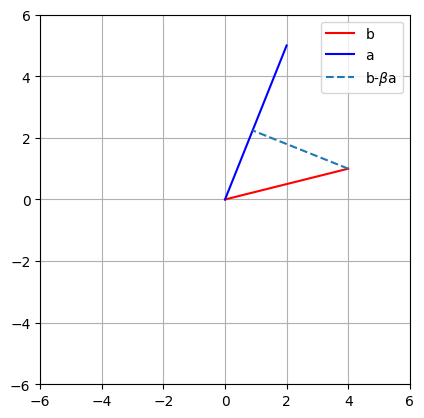
Projection Derivation#
Start with the condition for projection:
This states that the vector
Expand and rearrange:
Solve for
:
Thus, the scalar
Finally, the projection of
notice :
Projection methods in (vector to vector)#
Projection of Vector onto #
Method 1: Projection onto a Line#
The projection of
Steps:
Dot product of
:This step finds the magnitude of the projection of
in the direction of .Dot product of
:This calculates the magnitude of vector
, which helps in scaling the projection correctly.Scalar
:The ratio of the dot products gives the scalar that determines how much of
is in the direction of .Final projection:
The vector
is scaled by to get the projection.
Method 2: Projection Using a Unit Vector#
In this method, we first normalize
Steps:
Normalize
:This step ensures that the projection is independent of the magnitude of
, focusing only on the direction.Dot product of
:This calculates the component of
in the direction of the unit vector .Final projection:
The projection is obtained by scaling the unit vector
.
Method 3: Projection as a Linear Transformation#
Since projections are linear transformations, they can also be expressed as a matrix-vector multiplication.
Steps:
Unit vector
: From Method 2, the unit vector is:Construct the projection matrix
:This matrix represents the transformation that projects any vector onto the line defined by
.Matrix multiplication:
The projection is found by multiplying the matrix
by .
Final Result#
For all three methods, the projection of
These methods illustrate different approaches to the same problem, each confirming the correctness of the projection result.
Projection methods in implementation in python#
import numpy as np
import matplotlib.pyplot as plt
# Method1
def projection_onto_line(b, a):
dot_product_b_a = np.dot(b, a)
dot_product_a_a = np.dot(a, a)
beta = dot_product_b_a / dot_product_a_a
proj = beta * a
return proj
# Merhod2
def projection_using_unit_vector(b, a):
norm_a = np.linalg.norm(a)
u_hat = a / norm_a
dot_product_b_u_hat = np.dot(b, u_hat)
proj = dot_product_b_u_hat * u_hat
return proj
# Method3
def projection_as_matrix_product(b, a):
norm_a = np.linalg.norm(a)
u_hat = a / norm_a
A = np.array([[u_hat[0]**2, u_hat[0]*u_hat[1]],
[u_hat[0]*u_hat[1], u_hat[1]**2]])
proj = A @ b
return proj
def plot_projection(b, a, proj, method_name, ax):
# Plot original vectors and projection
ax.quiver(0, 0, b[0], b[1], angles='xy', scale_units='xy', scale=1, color='blue', label='Vector b', linewidth=2)
ax.quiver(0, 0, a[0], a[1], angles='xy', scale_units='xy', scale=1, color='red', label='Vector a', linewidth=2)
ax.quiver(0, 0, proj[0], proj[1], angles='xy', scale_units='xy', scale=1, color='green', label=f'Projection ({method_name})', linewidth=2, alpha=0.7)
# Plot projection line
ax.plot([b[0], proj[0]], [b[1], proj[1]], color='orange', linestyle='--', linewidth=1.5, alpha=0.5, label='b to Projection')
# Set limits and grid
ax.set_xlim(-1, 5)
ax.set_ylim(-1, 5)
ax.grid()
ax.axhline(0, color='black', linewidth=0.5, ls='--')
ax.axvline(0, color='black', linewidth=0.5, ls='--')
ax.set_aspect('equal', adjustable='box')
ax.set_title(f'Projection of Vector b onto Vector a - {method_name}')
ax.legend()
# Define the vectors
b = np.array([4, 1]) # Vector b
a = np.array([1, 4]) # Vector a
# Calculate projections
proj_line = projection_onto_line(b, a)
proj_unit_vector = projection_using_unit_vector(b, a)
proj_matrix_product = projection_as_matrix_product(b, a)
# Create a figure with subplots in one row
fig, axs = plt.subplots(1, 3, figsize=(18, 6))
# Plotting the projections
plot_projection(b, a, proj_line, "Line Projection", axs[0])
plot_projection(b, a, proj_unit_vector, "Unit Vector Projection", axs[1])
plot_projection(b, a, proj_matrix_product, "Matrix-Vector Product Projection", axs[2])
plt.tight_layout()
plt.show()
print(f"Method 1: {proj_line.round(2)}")
print(f"Method 2: {proj_unit_vector.round(2)}")
print(f"Method 3: {proj_matrix_product.round(2)}")
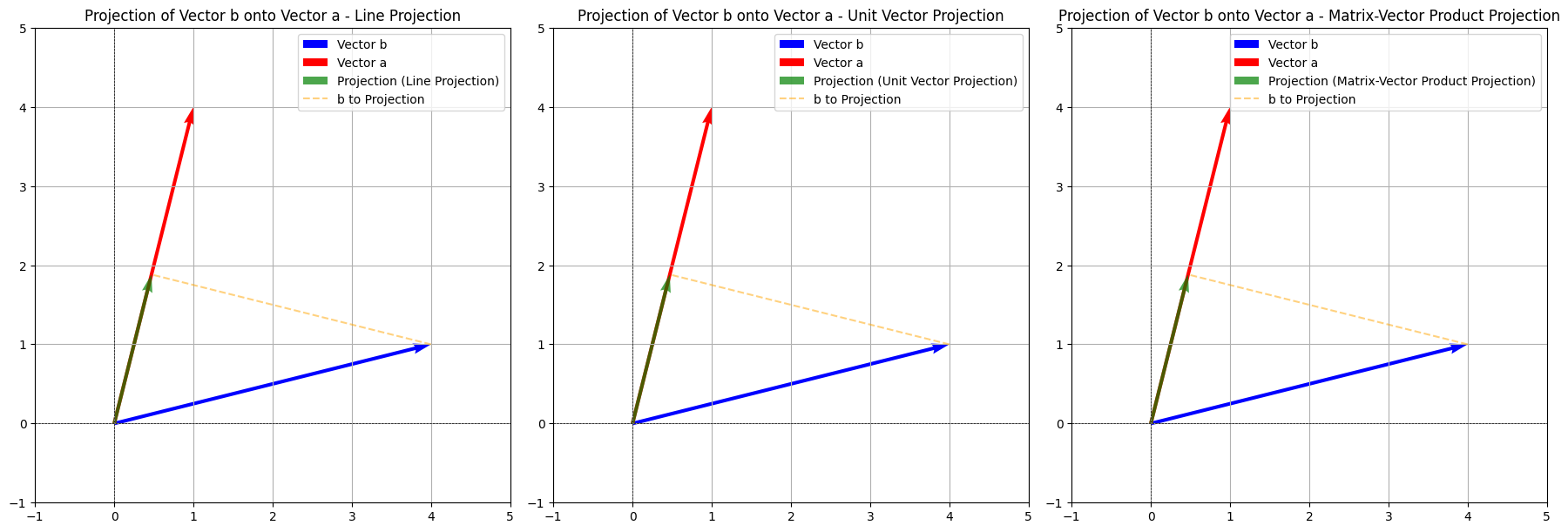
Method 1: [0.47 1.88]
Method 2: [0.47 1.88]
Method 3: [0.47 1.88]
Projection methods in (Vector to Vector)#
Projection of Vector onto #
Method 1: Projection onto a Line#
The projection of
Steps:#
Dot product
:
Dot product $
$:
Calculate scalar
:
Final projection:
Method 2: Projection Using a Unit Vector#
Normalize
:
Dot product
:
Final projection:
Method 3: Projection as a Matrix-Vector Product#
Unit vector
(as calculated earlier):
Construct the projection matrix ( A ):
Matrix multiplication:
Final Result#
For all three methods, the projection of
These methods confirm the correctness of the projection result in
Projection methods in implementation in python (Vector to Vector)#
import numpy as np
import matplotlib.pyplot as plt
from mpl_toolkits.mplot3d import Axes3D
# Define the vectors
b = np.array([4, 1, 2])
a = np.array([1, 4, 3])
# Method 1: Projection onto a Line
def projection_line(b, a):
return (np.dot(b, a) / np.dot(a, a)) * a
proj_line = projection_line(b, a)
# Method 2: Projection Using a Unit Vector
def projection_unit(b, a):
u = a / np.linalg.norm(a) # Normalize a
return np.dot(b, u) * u
proj_unit = projection_unit(b, a)
# Method 3: Projection as a Matrix-Vector Product
def projection_matrix(b, a):
u = a / np.linalg.norm(a) # Normalize a
projection_matrix = np.outer(u, u) # Outer product to form the matrix
return projection_matrix @ b # Matrix-vector multiplication
proj_matrix = projection_matrix(b, a)
# Prepare the figure
fig = plt.figure(figsize=(15, 5))
# Plot Method 1: Direct Projection
ax1 = fig.add_subplot(131, projection='3d')
ax1.quiver(0, 0, 0, b[0], b[1], b[2], color='r', label='Vector b', arrow_length_ratio=0.1)
ax1.quiver(0, 0, 0, a[0], a[1], a[2], color='g', label='Vector a', arrow_length_ratio=0.1)
ax1.quiver(0, 0, 0, proj_line[0], proj_line[1], proj_line[2], color='b', label='Projection', arrow_length_ratio=0.1)
# Dashed line from b to the projection point
ax1.plot([b[0], proj_line[0]], [b[1], proj_line[1]], [b[2], proj_line[2]], 'k--', label='Line to Projection')
ax1.set_xlim([-1, 5])
ax1.set_ylim([-1, 5])
ax1.set_zlim([-1, 5])
ax1.set_title('Projection onto a Line')
ax1.set_xlabel('X axis')
ax1.set_ylabel('Y axis')
ax1.set_zlabel('Z axis')
ax1.legend()
# Plot Method 2: Using a Unit Vector
ax2 = fig.add_subplot(132, projection='3d')
ax2.quiver(0, 0, 0, b[0], b[1], b[2], color='r', label='Vector b', arrow_length_ratio=0.1)
ax2.quiver(0, 0, 0, a[0], a[1], a[2], color='g', label='Vector a', arrow_length_ratio=0.1)
ax2.quiver(0, 0, 0, proj_unit[0], proj_unit[1], proj_unit[2], color='b', label='Projection', arrow_length_ratio=0.1)
# Dashed line from b to the projection point
ax2.plot([b[0], proj_unit[0]], [b[1], proj_unit[1]], [b[2], proj_unit[2]], 'k--', label='Line to Projection')
ax2.set_xlim([-1, 5])
ax2.set_ylim([-1, 5])
ax2.set_zlim([-1, 5])
ax2.set_title('Projection using a Unit Vector')
ax2.set_xlabel('X axis')
ax2.set_ylabel('Y axis')
ax2.set_zlabel('Z axis')
ax2.legend()
# Plot Method 3: Matrix-Vector Product
ax3 = fig.add_subplot(133, projection='3d')
ax3.quiver(0, 0, 0, b[0], b[1], b[2], color='r', label='Vector b', arrow_length_ratio=0.1)
ax3.quiver(0, 0, 0, a[0], a[1], a[2], color='g', label='Vector a', arrow_length_ratio=0.1)
ax3.quiver(0, 0, 0, proj_matrix[0], proj_matrix[1], proj_matrix[2], color='b', label='Projection', arrow_length_ratio=0.1)
# Dashed line from b to the projection point
ax3.plot([b[0], proj_matrix[0]], [b[1], proj_matrix[1]], [b[2], proj_matrix[2]], 'k--', label='Line to Projection')
ax3.set_xlim([-1, 5])
ax3.set_ylim([-1, 5])
ax3.set_zlim([-1, 5])
ax3.set_title('Projection using Matrix-Vector Product')
ax3.set_xlabel('X axis')
ax3.set_ylabel('Y axis')
ax3.set_zlabel('Z axis')
ax3.legend()
# Show the plots
plt.tight_layout()
plt.show()
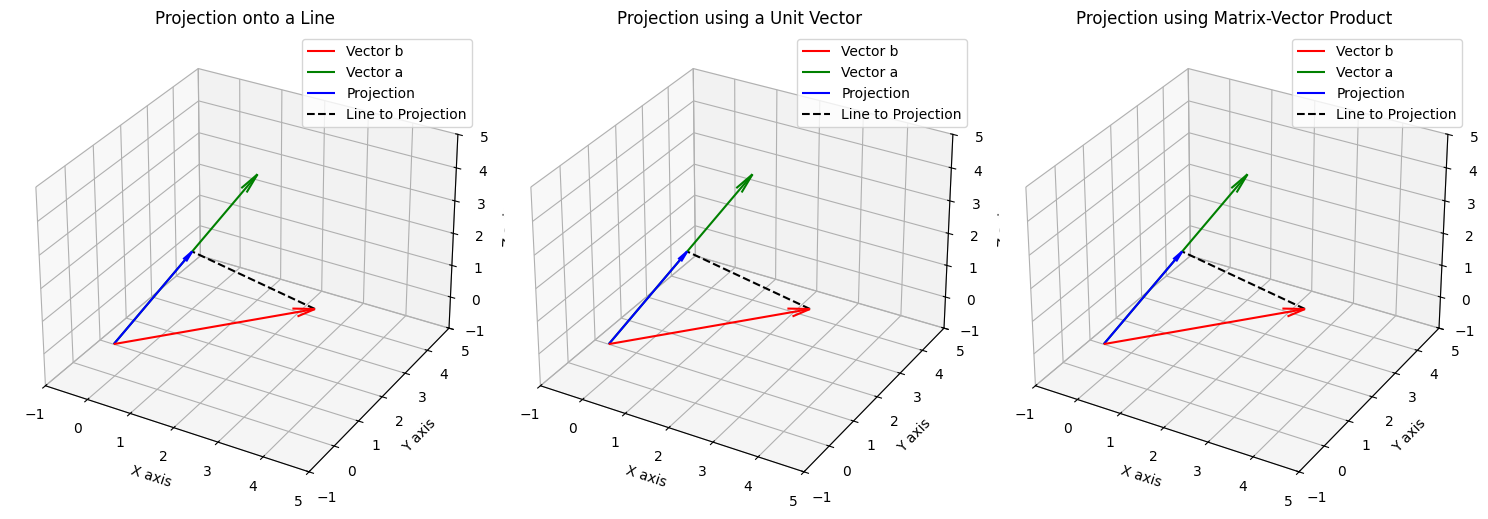
Definition of Projection in #
The projection of a vector
Mathematical Expression:#
The projection of
where:
is the Gram matrix, representing the inner products of the columns of , is the inverse of the Gram matrix (if it exists), is the projection of onto the rows of .
Geometric Interpretation:#
The resulting vector
Key Characteristics:#
The projection minimizes the distance between
and the column space of .The residual
is orthogonal to the column space of .This method is used in solving least squares problems, where the system
may have no exact solution due to being overdetermined (more equations than unknowns).
Applications:#
Least squares regression, fitting a model to data points.
Dimensionality reduction, projecting high-dimensional data into a lower-dimensional subspace.