GloVe: Word Representation#
Author : Seyed Rasul Mortazavi Moghadam
Contact : s.rasul.mortazavi@gmail.com
What is GloVe?#
GloVe (Global Vectors for word representation), is a model for creating word embeddings that encodes both the meaning of words and their relationships with other words in the same vector space. The core idea behind GloVe is that the co-occurrence of words in a text can provide valuable information about their meanings. If two words frequently appear together (e.g., βkingβ and βqueenβ), then their meanings should be closely related. GloVe uses this co-occurrence information to generate vector representations of words that reflect these relationships.
GloVe encode the co-occurrence probability ratio between two words as vector differences. GloVe uses a weighted least squares objective that minimizes the difference between the dot product of the vectors of two words and the logarithm of their number of co-occurrences:
$\(J=\sum_{i, j=1}^{V}f\left(π_{i j}\right)(w^{T}_{i}\tilde{w}_{j} + b_{i} + \tilde{b}_{j} - \log{π}_{ij})^{2}\)$
where \(w_{i}\) and \(b_{i}\) are the word vector and bias respectively of word \({i}\), \(w^{T}_{i}\) and \({b}_{j}\) are the context word vector and bias respectively of word \({j}\), \({π}_{ij}\) is the number of times word \({i}\) occurs in the context of word \({j}\) (the values present in the Co-occurrence matrix), and \(f\) is a weighting function to reduce the impact of very frequent word pairs, such as stop words (e.g., βthe,β βandβ). It assigns lower weights to very frequent co-occurrences. The weighting function \(f(x)\) is designed to reduce the influence of very high \(x\) values, while still preserving important co-occurrence information.
The weighting function is typically defined as: $\(f(x) = \begin{cases} (\dfrac{x}{x_{max}})^\alpha & \quad \text{\){x} < x_{max}\(}\\ 1 & \quad \text{otherwise} \end{cases}\)$
where \(\alpha\) is a tuning parameter and \(x_{max}\) is a threshold.
Example: For very frequent words like βtheβ or βon,β even if they co-occur often, their influence on the vector training is reduced.
# @hidden_cell
import numpy as np
import matplotlib.pyplot as plt
xmax = 100
alpha = 3/4
x = np.linspace(0, 300, 500)
y = np.where(x < xmax, (x / xmax)**alpha, 1)
plt.plot(x, y, label=f'alpha = {alpha}, xmax = {xmax}' , linewidth=3)
plt.axhline(1, linestyle='--', color='gray', linewidth=0.5) # horizontal lines
plt.axvline(100, linestyle='--', color='gray', linewidth=0.5) # vertical lines
plt.title('Weighting Function f(x)')
plt.xlabel('x')
plt.ylabel('f(x)')
plt.legend()
plt.show()
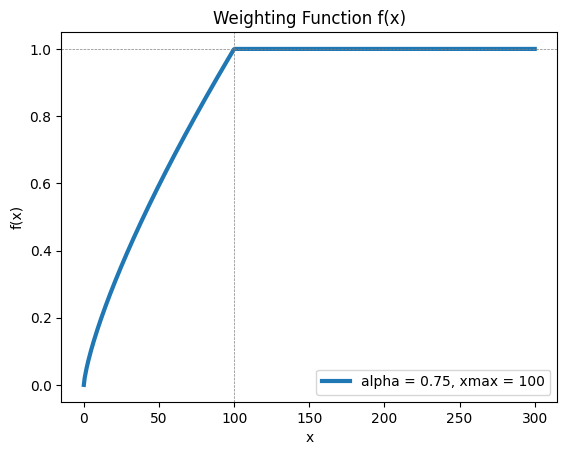
Why Do We Need GloVe?#
Computers canβt directly understand words or text. They require numerical representations to process them. Early methods like Bag of Words and TF-IDF (Term Frequency-Inverse Document Frequency) were used to represent text as vectors, but they had significant limitations:
They didnβt capture the meaning of words effectively.
Words with similar meanings were represented very differently.
There was no understanding of relationships between words, such as βcatβ and βkitten.β
To overcome these limitations, new methods like Word2Vec and GloVe were introduced to generate word embeddings, where each word is represented as a vector in a way that captures semantic relationships.
How Does GloVe Work?#
1: Building a Co-occurrence Matrix:#
GloVe starts by analyzing a large text corpus and creating a co-occurrence matrix. This matrix counts how frequently each word appears in the context of other words. The size of the context window (e.g., 5 words before and after) is defined.
Example: Consider the following sentences:
βThe cat sits on the mat.β
βThe dog lies on the mat.β
We build a co-occurrence matrix where each cell \(π_{i j}\) represents how often word \(j\) appears in the context of word \(i\). co-occurrence matrix:
the |
cat |
sits |
on |
mat |
dog |
lies |
|
---|---|---|---|---|---|---|---|
the |
0 |
2 |
1 |
2 |
2 |
1 |
1 |
cat |
2 |
0 |
1 |
1 |
1 |
0 |
0 |
sits |
1 |
1 |
0 |
1 |
1 |
0 |
0 |
on |
2 |
1 |
1 |
0 |
2 |
1 |
1 |
mat |
2 |
1 |
1 |
2 |
0 |
1 |
1 |
dog |
1 |
0 |
0 |
1 |
1 |
0 |
1 |
lies |
1 |
0 |
0 |
1 |
1 |
1 |
0 |
In this matrix, for instance, βcatβ appears in the context of βtheβ 2 times, and βonβ appears with βtheβ 2 times, and so on.
After the values are weighted based on the proximity or distance of the words from each other. This is an example representation; the actual weights would depend on how you define and calculate them based on your specific application (e.g., distance in terms of number of words, etc.).
Weighted Co-occurrence Matrix:
the |
cat |
sits |
on |
mat |
dog |
lies |
|
---|---|---|---|---|---|---|---|
the |
0 |
1.5 |
1 |
2 |
1.5 |
1 |
1 |
cat |
1.5 |
0 |
1 |
1 |
1 |
0 |
0 |
sits |
1 |
1 |
0 |
1 |
1 |
0 |
0 |
on |
2 |
1 |
1 |
0 |
2 |
1 |
1 |
mat |
1.5 |
1 |
1 |
2 |
0 |
1 |
1 |
dog |
1 |
0 |
0 |
1 |
1 |
0 |
1 |
lies |
1 |
0 |
0 |
1 |
1 |
1 |
0 |
2: Capturing Relationships Between Words:#
The co-occurrence matrix captures how words are related. For example, βappleβ and βfruitβ might appear together frequently, so the matrix would reflect that connection. Conversely, βappleβ and βcarβ might not appear together often, so their relationship is weaker.
3: Learning Word Vectors:#
GloVe uses the co-occurrence information to train word vectors. The idea is that two words that often appear together should have similar embeddings. The model tries to optimize the vectors so that the dot product of two word vectors reflects how often those words co-occur.
4: Optimization with a Loss Function:#
GloVe minimizes a loss function that measures how well the word embeddings predict the word co-occurrence information. The goal is to make sure that word vectors capture the relationships between words by preserving the global co-occurrence patterns. This process is done iteratively, adjusting the vectors after each iteration until it reaches a satisfactory solution.
5: Word Vectors as Final Output:#
After training, GloVe produces word embeddings: vectors that represent each word. These vectors can then be used for various NLP tasks, such as text classification, sentiment analysis, and machine translation.
Practice with code#
To feed the GloVe algorithm, here we use Telegram, we scrapes posts of a public channel using Telegram web preview.(in this example, we scrape Telegramβs own notification channel).
Limitations:
Pagination and More Posts: This code only scrapes posts on the current page whitout pagination.
Multimedia Posts: This code only extracts text content of the posts(not images, videos, β¦)
Telegram Restrictions: If the channel is private or requires login, this method wonβt work, since it only scrapes public content available on the web.
Get posts from Telegram:#
Fetching the Web Page:
We first make a request to the web version of the Telegram channel to get the HTML content of the page.
Parsing the Page:
The HTML content is then parsed using BeautifulSoup, allowing us to navigate through the HTML tree structure and locate the relevant elements (i.e., the posts).
Extracting the Post Content:
All the posts are contained within < div > elements that share a specific class (tgme_widget_message_text). We extract all of these elements and loop through them to print the post content.
Make sure that your internet connection is established and your IP address is not from Iran. You can install required libraries using using the command below if you donβt have them already:
pip install requests beautifulsoup4
Requirement already satisfied: requests in h:\hadisadoghiyazdi\circuit\lib\site-packages (2.32.3)
Requirement already satisfied: beautifulsoup4 in h:\hadisadoghiyazdi\circuit\lib\site-packages (4.12.3)
Requirement already satisfied: charset-normalizer<4,>=2 in h:\hadisadoghiyazdi\circuit\lib\site-packages (from requests) (3.4.0)
Requirement already satisfied: idna<4,>=2.5 in h:\hadisadoghiyazdi\circuit\lib\site-packages (from requests) (3.10)
Requirement already satisfied: urllib3<3,>=1.21.1 in h:\hadisadoghiyazdi\circuit\lib\site-packages (from requests) (2.2.3)
Requirement already satisfied: certifi>=2017.4.17 in h:\hadisadoghiyazdi\circuit\lib\site-packages (from requests) (2024.8.30)
Requirement already satisfied: soupsieve>1.2 in h:\hadisadoghiyazdi\circuit\lib\site-packages (from beautifulsoup4) (2.6)
Note: you may need to restart the kernel to use updated packages.
# Importing Required Libraries:
# requests: This is a Python library used for sending HTTP requests. We use it to fetch the HTML content of the Telegram web preview.
# BeautifulSoup: from the bs4 module: This is used for parsing the HTML content and extracting the relevant information (in this case, the posts).
import requests
from bs4 import BeautifulSoup
# URL of the Public Channel:
# The URL points to the Telegram channel's web preview page, where posts are visible to users who don't have the app.
# URL of the Telegram channel preview page (https://t.me/s/CHANNLE_NAME)
channel_url = 'https://t.me/s/telegram'
# a GET request to fetch the channel page
response = requests.get(channel_url)
# check we get a successful response
response.raise_for_status()
# Parsing the HTML Content of the page with BeautifulSoup
soup = BeautifulSoup(response.content, 'lxml')
# Finding All the Posts
# We need to locate all the HTML elements that contain the post content.
# On the Telegram web preview, post texts are typically contained within <div> elements that have the class tgme_widget_message_text.
# We use soup.find_all() to find all these <div> elements.
def get_posts(soup):
posts = []
# Find all the divs containing the posts
posts_text = soup.find_all('div', class_='tgme_widget_message_text')
for post_text in posts_text:
# Check the post contains text
if post_text:
post_content = post_text.get_text(separator="\n").strip()
posts.append(post_content)
posts.reverse()
return posts
posts = get_posts(soup)
#Corpus using in the next step
corpus = []
#Split each post by space into an array
for idx, post in enumerate(posts, 1):
corpus.append(post.split())
print(*corpus, sep='\n')
---------------------------------------------------------------------------
OSError Traceback (most recent call last)
File H:\HadiSadoghiYazdi\Circuit\Lib\site-packages\urllib3\connection.py:199, in HTTPConnection._new_conn(self)
198 try:
--> 199 sock = connection.create_connection(
200 (self._dns_host, self.port),
201 self.timeout,
202 source_address=self.source_address,
203 socket_options=self.socket_options,
204 )
205 except socket.gaierror as e:
File H:\HadiSadoghiYazdi\Circuit\Lib\site-packages\urllib3\util\connection.py:85, in create_connection(address, timeout, source_address, socket_options)
84 try:
---> 85 raise err
86 finally:
87 # Break explicitly a reference cycle
File H:\HadiSadoghiYazdi\Circuit\Lib\site-packages\urllib3\util\connection.py:73, in create_connection(address, timeout, source_address, socket_options)
72 sock.bind(source_address)
---> 73 sock.connect(sa)
74 # Break explicitly a reference cycle
OSError: [WinError 10051] A socket operation was attempted to an unreachable network
The above exception was the direct cause of the following exception:
NewConnectionError Traceback (most recent call last)
File H:\HadiSadoghiYazdi\Circuit\Lib\site-packages\urllib3\connectionpool.py:789, in HTTPConnectionPool.urlopen(self, method, url, body, headers, retries, redirect, assert_same_host, timeout, pool_timeout, release_conn, chunked, body_pos, preload_content, decode_content, **response_kw)
788 # Make the request on the HTTPConnection object
--> 789 response = self._make_request(
790 conn,
791 method,
792 url,
793 timeout=timeout_obj,
794 body=body,
795 headers=headers,
796 chunked=chunked,
797 retries=retries,
798 response_conn=response_conn,
799 preload_content=preload_content,
800 decode_content=decode_content,
801 **response_kw,
802 )
804 # Everything went great!
File H:\HadiSadoghiYazdi\Circuit\Lib\site-packages\urllib3\connectionpool.py:490, in HTTPConnectionPool._make_request(self, conn, method, url, body, headers, retries, timeout, chunked, response_conn, preload_content, decode_content, enforce_content_length)
489 new_e = _wrap_proxy_error(new_e, conn.proxy.scheme)
--> 490 raise new_e
492 # conn.request() calls http.client.*.request, not the method in
493 # urllib3.request. It also calls makefile (recv) on the socket.
File H:\HadiSadoghiYazdi\Circuit\Lib\site-packages\urllib3\connectionpool.py:466, in HTTPConnectionPool._make_request(self, conn, method, url, body, headers, retries, timeout, chunked, response_conn, preload_content, decode_content, enforce_content_length)
465 try:
--> 466 self._validate_conn(conn)
467 except (SocketTimeout, BaseSSLError) as e:
File H:\HadiSadoghiYazdi\Circuit\Lib\site-packages\urllib3\connectionpool.py:1095, in HTTPSConnectionPool._validate_conn(self, conn)
1094 if conn.is_closed:
-> 1095 conn.connect()
1097 # TODO revise this, see https://github.com/urllib3/urllib3/issues/2791
File H:\HadiSadoghiYazdi\Circuit\Lib\site-packages\urllib3\connection.py:693, in HTTPSConnection.connect(self)
692 sock: socket.socket | ssl.SSLSocket
--> 693 self.sock = sock = self._new_conn()
694 server_hostname: str = self.host
File H:\HadiSadoghiYazdi\Circuit\Lib\site-packages\urllib3\connection.py:214, in HTTPConnection._new_conn(self)
213 except OSError as e:
--> 214 raise NewConnectionError(
215 self, f"Failed to establish a new connection: {e}"
216 ) from e
218 # Audit hooks are only available in Python 3.8+
NewConnectionError: <urllib3.connection.HTTPSConnection object at 0x000001B824C36270>: Failed to establish a new connection: [WinError 10051] A socket operation was attempted to an unreachable network
The above exception was the direct cause of the following exception:
MaxRetryError Traceback (most recent call last)
File H:\HadiSadoghiYazdi\Circuit\Lib\site-packages\requests\adapters.py:667, in HTTPAdapter.send(self, request, stream, timeout, verify, cert, proxies)
666 try:
--> 667 resp = conn.urlopen(
668 method=request.method,
669 url=url,
670 body=request.body,
671 headers=request.headers,
672 redirect=False,
673 assert_same_host=False,
674 preload_content=False,
675 decode_content=False,
676 retries=self.max_retries,
677 timeout=timeout,
678 chunked=chunked,
679 )
681 except (ProtocolError, OSError) as err:
File H:\HadiSadoghiYazdi\Circuit\Lib\site-packages\urllib3\connectionpool.py:843, in HTTPConnectionPool.urlopen(self, method, url, body, headers, retries, redirect, assert_same_host, timeout, pool_timeout, release_conn, chunked, body_pos, preload_content, decode_content, **response_kw)
841 new_e = ProtocolError("Connection aborted.", new_e)
--> 843 retries = retries.increment(
844 method, url, error=new_e, _pool=self, _stacktrace=sys.exc_info()[2]
845 )
846 retries.sleep()
File H:\HadiSadoghiYazdi\Circuit\Lib\site-packages\urllib3\util\retry.py:519, in Retry.increment(self, method, url, response, error, _pool, _stacktrace)
518 reason = error or ResponseError(cause)
--> 519 raise MaxRetryError(_pool, url, reason) from reason # type: ignore[arg-type]
521 log.debug("Incremented Retry for (url='%s'): %r", url, new_retry)
MaxRetryError: HTTPSConnectionPool(host='t.me', port=443): Max retries exceeded with url: /s/telegram (Caused by NewConnectionError('<urllib3.connection.HTTPSConnection object at 0x000001B824C36270>: Failed to establish a new connection: [WinError 10051] A socket operation was attempted to an unreachable network'))
During handling of the above exception, another exception occurred:
ConnectionError Traceback (most recent call last)
Cell In[3], line 17
13 channel_url = 'https://t.me/s/telegram'
16 # a GET request to fetch the channel page
---> 17 response = requests.get(channel_url)
19 # check we get a successful response
20 response.raise_for_status()
File H:\HadiSadoghiYazdi\Circuit\Lib\site-packages\requests\api.py:73, in get(url, params, **kwargs)
62 def get(url, params=None, **kwargs):
63 r"""Sends a GET request.
64
65 :param url: URL for the new :class:`Request` object.
(...)
70 :rtype: requests.Response
71 """
---> 73 return request("get", url, params=params, **kwargs)
File H:\HadiSadoghiYazdi\Circuit\Lib\site-packages\requests\api.py:59, in request(method, url, **kwargs)
55 # By using the 'with' statement we are sure the session is closed, thus we
56 # avoid leaving sockets open which can trigger a ResourceWarning in some
57 # cases, and look like a memory leak in others.
58 with sessions.Session() as session:
---> 59 return session.request(method=method, url=url, **kwargs)
File H:\HadiSadoghiYazdi\Circuit\Lib\site-packages\requests\sessions.py:589, in Session.request(self, method, url, params, data, headers, cookies, files, auth, timeout, allow_redirects, proxies, hooks, stream, verify, cert, json)
584 send_kwargs = {
585 "timeout": timeout,
586 "allow_redirects": allow_redirects,
587 }
588 send_kwargs.update(settings)
--> 589 resp = self.send(prep, **send_kwargs)
591 return resp
File H:\HadiSadoghiYazdi\Circuit\Lib\site-packages\requests\sessions.py:703, in Session.send(self, request, **kwargs)
700 start = preferred_clock()
702 # Send the request
--> 703 r = adapter.send(request, **kwargs)
705 # Total elapsed time of the request (approximately)
706 elapsed = preferred_clock() - start
File H:\HadiSadoghiYazdi\Circuit\Lib\site-packages\requests\adapters.py:700, in HTTPAdapter.send(self, request, stream, timeout, verify, cert, proxies)
696 if isinstance(e.reason, _SSLError):
697 # This branch is for urllib3 v1.22 and later.
698 raise SSLError(e, request=request)
--> 700 raise ConnectionError(e, request=request)
702 except ClosedPoolError as e:
703 raise ConnectionError(e, request=request)
ConnectionError: HTTPSConnectionPool(host='t.me', port=443): Max retries exceeded with url: /s/telegram (Caused by NewConnectionError('<urllib3.connection.HTTPSConnection object at 0x000001B824C36270>: Failed to establish a new connection: [WinError 10051] A socket operation was attempted to an unreachable network'))
We have prepared the corpus up to this stage, now letβs go to the implementation of the glove algorithm.
1. Import Required Libraries:#
numpy: Used for numerical operations like matrix and vector calculations.
collections.Counter: A specialized dictionary for counting occurrences of elements.
collections.defaultdict: A dictionary that provides a default value for non-existing keys.
tqdm: A library to show a progress bar during iteration (useful during training).
itertools: Helps in working with iterators for efficient looping.
matplotlib.pyplot: This library is used for plotting graphs in Python.
import numpy as np
from collections import Counter, defaultdict
from tqdm import tqdm
import itertools
import matplotlib.pyplot as plt
To install required libraries:
pip install numpy collections tqdm itertools matplotlib
2. Step 1: Prepare Data and Build the Co-occurrence Matrix#
The first step in GloVe is to create a co-occurrence matrix, which captures how often words appear together within a given window size in the corpus.
Input: The corpus (list of tokenized sentences) and window_size (window_size=5 means we consider the 5 words before and after the target word as its context).
Vocabulary: We extract all unique words from the corpus and assign each word a unique ID (word_to_id).
Co-occurrence Matrix: For each word in a sentence, we count how often other words appear within its context window. The weight of the context word is inversely proportional to the distance from the target word.
# Step1: prepare data and build co-occurrence matrix
def build_cooccurrence_matrix(corpus, window_size=5):
vocab = set(itertools.chain(*corpus))
word_to_id = {word: i for i, word in enumerate(vocab)}
id_to_word = {i: word for word, i in word_to_id.items()}
cooccurrence_matrix = defaultdict(Counter)
for sentence in corpus:
for i, word in enumerate(sentence):
word_id = word_to_id[word]
start = max(0, i - window_size)
end = min(len(sentence), i + window_size + 1)
for j in range(start, end):
if i != j:
context_word = sentence[j]
context_word_id = word_to_id[context_word]
cooccurrence_matrix[word_id][context_word_id] += 1 / abs(j - i) # weighted by distance
return cooccurrence_matrix, word_to_id, id_to_word
3. GloVe Loss Function#
This function computes the loss for each word pair in the GloVe model.
wi and wj: The embedding vectors for the word and its context word.
bi and bj: The biases for the word and its context word.
Xij: The co-occurrence count between the word and context word.
X_max and alpha: Hyperparameters used to weight the co-occurrence counts.
The loss function minimizes the difference between the dot product of the word and context embeddings and the logarithm of the co-occurrence count, adjusted by a weighting function.
# GloVe loss function
def glove_loss(wi, wj, bi, bj, Xij, X_max, alpha):
weighting = (Xij / X_max)**alpha if Xij < X_max else 1
loss = weighting * (wi.dot(wj) + bi + bj - np.log(Xij))**2
return loss
4. Training the GloVe Model#
This function optimizes the word and context embeddings using gradient descent.
Parameters:
embedding_dim: The dimension of the word embeddings.
iterations: The number of training epochs.
learning_rate: The step size for the gradient descent.
X_max and alpha: Control how much weight each co-occurrence count receives.
Initialization:
Word and context embeddings (W, W_context) are initialized randomly.
Bias terms (biases, biases_context) are initialized to zeros.
Training Loop:
For each epoch, the algorithm iterates over each word-context pair in the co-occurrence matrix.
Loss is calculated using the βglove_lossβ function, and gradients are computed for each parameter.
Parameters (W, W_context, biases, and biases_context) are updated using gradient descent.
Progress is tracked using βtqdmβ, and total loss is printed after each epoch.
# Optimize parameters
def train_glove(cooccurrence_matrix, word_to_id, embedding_dim=50, iterations=100, learning_rate=0.05, X_max=100, alpha=0.75):
vocab_size = len(word_to_id)
# GloVe Model parameters
W = np.random.randn(vocab_size, embedding_dim) / np.sqrt(vocab_size)
W_context = np.random.randn(vocab_size, embedding_dim) / np.sqrt(vocab_size)
biases = np.zeros(vocab_size)
biases_context = np.zeros(vocab_size)
loss_list = []
# Training
for epoch in range(iterations):
total_loss = 0
for word_id, context in tqdm(cooccurrence_matrix.items(), desc=f'Epoch {epoch+1}'):
for context_word_id, Xij in context.items():
# main word and context word
wi = W[word_id]
wj = W_context[context_word_id]
bi = biases[word_id]
bj = biases_context[context_word_id]
# Calculate loss
loss = glove_loss(wi, wj, bi, bj, Xij, X_max, alpha)
total_loss += loss
# Gradient descent for each parameter
weighting = (Xij / X_max)**alpha if Xij < X_max else 1
grad_wi = weighting * (wi.dot(wj) + bi + bj - np.log(Xij)) * wj
grad_wj = weighting * (wi.dot(wj) + bi + bj - np.log(Xij)) * wi
grad_bi = weighting * (wi.dot(wj) + bi + bj - np.log(Xij))
grad_bj = weighting * (wi.dot(wj) + bi + bj - np.log(Xij))
# Update parameters
W[word_id] -= learning_rate * grad_wi
W_context[context_word_id] -= learning_rate * grad_wj
biases[word_id] -= learning_rate * grad_bi
biases_context[context_word_id] -= learning_rate * grad_bj
loss_list.append(total_loss)
return W, W_context, loss_list
# Building the Co-occurrence Matrix
cooccurrence_matrix, word_to_id, id_to_word = build_cooccurrence_matrix(corpus)
embedding_dim = 50 # Length of each word vector
iterations = 100 # Number of epochs
# The GloVe model is trained for 100 iterations with a vector dimension of 50.
W, W_context, loss_list = train_glove(cooccurrence_matrix, word_to_id, embedding_dim, iterations)
# Plot Chart of loss by epoches
plt.plot( loss_list, label=f'Vocabulary size: {len(word_to_id)}' , linewidth=2, color='red')
plt.title('Loss by epoch')
plt.xlabel('epoch')
plt.ylabel('Loss')
plt.legend()
plt.show()
word = 'Telegram'
word_id = word_to_id[word]
word_vector = W[word_id]
# Show vector of an example word : "telegram"
print(f"Vector of "'{word}'" word:\n{word_vector}, word_id:{word_id}")
# Plot the word vector as a bar chart
plt.figure(figsize=(10, 6))
plt.bar(range(len(word_vector)), word_vector)
plt.title(f'Word Vector for "{word}"')
plt.xlabel('Vector Component Index')
plt.ylabel('Value')
plt.show()
All code sections in one:#
import requests
from bs4 import BeautifulSoup
import numpy as np
from collections import Counter, defaultdict
from tqdm import tqdm
import itertools
import matplotlib.pyplot as plt
# URL of the Telegram channel preview page (https://t.me/s/CHANNLE_NAME)
channel_url = 'https://t.me/s/telegram'
# a GET request to fetch the channel page
response = requests.get(channel_url)
# check we get a successful response
response.raise_for_status()
# Parsing the HTML Content of the page with BeautifulSoup
soup = BeautifulSoup(response.content, 'lxml')
# Finding All the Posts
def get_posts(soup):
posts = []
# Find all the divs containing the posts
posts_text = soup.find_all('div', class_='tgme_widget_message_text')
for post_text in posts_text:
# Check the post contains text
if post_text:
post_content = post_text.get_text(separator="\n").strip()
posts.append(post_content)
posts.reverse()
return posts
posts = get_posts(soup)
#Corpus using in the next step
corpus = []
#Split each post by space into an array
for idx, post in enumerate(posts, 1):
corpus.append(post.split())
print(*corpus, sep='\n')
# Step1: prepare data and build co-occurrence matrix
def build_cooccurrence_matrix(corpus, window_size=5):
vocab = set(itertools.chain(*corpus))
word_to_id = {word: i for i, word in enumerate(vocab)}
id_to_word = {i: word for word, i in word_to_id.items()}
cooccurrence_matrix = defaultdict(Counter)
for sentence in corpus:
for i, word in enumerate(sentence):
word_id = word_to_id[word]
start = max(0, i - window_size)
end = min(len(sentence), i + window_size + 1)
for j in range(start, end):
if i != j:
context_word = sentence[j]
context_word_id = word_to_id[context_word]
cooccurrence_matrix[word_id][context_word_id] += 1 / abs(j - i) # weighted by distance
return cooccurrence_matrix, word_to_id, id_to_word
# GloVe loss function
def glove_loss(wi, wj, bi, bj, Xij, X_max, alpha):
weighting = (Xij / X_max)**alpha if Xij < X_max else 1
loss = weighting * (wi.dot(wj) + bi + bj - np.log(Xij))**2
return loss
# Optimize parameters
def train_glove(cooccurrence_matrix, word_to_id, embedding_dim=50, iterations=100, learning_rate=0.05, X_max=100, alpha=0.75):
vocab_size = len(word_to_id)
# GloVe Model parameters
W = np.random.randn(vocab_size, embedding_dim) / np.sqrt(vocab_size)
W_context = np.random.randn(vocab_size, embedding_dim) / np.sqrt(vocab_size)
biases = np.zeros(vocab_size)
biases_context = np.zeros(vocab_size)
loss_list = []
# Training
for epoch in range(iterations):
total_loss = 0
for word_id, context in tqdm(cooccurrence_matrix.items(), desc=f'Epoch {epoch+1}'):
for context_word_id, Xij in context.items():
# main word and context word
wi = W[word_id]
wj = W_context[context_word_id]
bi = biases[word_id]
bj = biases_context[context_word_id]
# Calculate loss
loss = glove_loss(wi, wj, bi, bj, Xij, X_max, alpha)
total_loss += loss
# Gradient descent for each parameter
weighting = (Xij / X_max)**alpha if Xij < X_max else 1
grad_wi = weighting * (wi.dot(wj) + bi + bj - np.log(Xij)) * wj
grad_wj = weighting * (wi.dot(wj) + bi + bj - np.log(Xij)) * wi
grad_bi = weighting * (wi.dot(wj) + bi + bj - np.log(Xij))
grad_bj = weighting * (wi.dot(wj) + bi + bj - np.log(Xij))
# Update parameters
W[word_id] -= learning_rate * grad_wi
W_context[context_word_id] -= learning_rate * grad_wj
biases[word_id] -= learning_rate * grad_bi
biases_context[context_word_id] -= learning_rate * grad_bj
loss_list.append(total_loss)
return W, W_context, loss_list
# Building the Co-occurrence Matrix
cooccurrence_matrix, word_to_id, id_to_word = build_cooccurrence_matrix(corpus)
embedding_dim = 50 # Length of each word vector
iterations = 100 # Number of epochs
# The GloVe model is trained for 100 iterations with a vector dimension of 50.
W, W_context, loss_list = train_glove(cooccurrence_matrix, word_to_id, embedding_dim, iterations)
# Plot Chart of loss by epoches
plt.plot( loss_list, label=f'Vocabulary size: {len(word_to_id)}' , linewidth=2, color='red')
plt.title('Loss by epoch')
plt.xlabel('epoch')
plt.ylabel('Loss')
plt.legend()
plt.show()
word = 'Telegram'
word_id = word_to_id[word]
word_vector = W[word_id]
# Show vector of an example word : "telegram"
print(f"Vector of \"'{word}'\" word :\n{word_vector}, word_id:{word_id}")
# Plot the word vector as a bar chart
plt.figure(figsize=(10, 6))
plt.bar(range(len(word_vector)), word_vector)
plt.title(f'Word Vector for "{word}"')
plt.xlabel('Vector Component Index')
plt.ylabel('Value')
plt.show()
['For', 'more', 'details', 'on', 'all', 'the', 'features', 'from', 'this', 'update,', 'check', 'out', 'our', 'latest', 'blog:', 'https://telegram.org/blog/gifts-verification-platform', 'October', 'Features', '1', 'β’', '2', 'β’', '3', 'β’', '4', 'β’', 'More']
['RTMP', 'Streams', 'on', 'Android.', 'Telegram', 'for', 'Android', 'now', 'supports', 'livestreaming', 'with', 'RTMP', '.', 'This', 'allows', 'users', 'to', 'generate', 'a', 'stream', 'key', 'and', 'connect', 'the', 'video', 'feed', 'to', 'popular', 'apps', '.', 'October', 'Features', '1', 'β’', '2', 'β’', '3', 'β’', '4', 'β’', 'More']
['Improved', 'Reporting', 'Interface.', 'The', 'reporting', 'menu', 'has', 'been', 'expanded', 'with', 'detailed', 'options', 'for', 'each', 'category.', 'The', 'list', 'of', 'reporting', 'reasons', 'can', 'now', 'update', 'dynamically', 'β', 'further', 'improving', 'reports', 'for', 'moderators', 'and', 'helping', 'scale', "Telegram's", 'AI', 'moderation', 'tools', '.', 'October', 'Features', '1', 'β’', '2', 'β’', '3', 'β’', '4', 'β’', 'More']
['Verification', 'Platform.', 'Any', 'business,', 'app', 'or', 'website', 'can', 'now', 'use', 'Telegram', 'to', 'send', 'verification', 'codes', 'to', 'their', 'users', 'β', 'for', 'just', '$0.01', 'per', 'message', '.', 'Telegram', 'codes', 'are', 'also', 'faster,', 'more', 'secure', 'and', 'more', 'reliable', 'than', 'other', 'alternatives.', 'Interested', 'developers', 'can', 'learn', 'more', 'in', 'our', 'detailed', 'guide', '.', 'October', 'Features', '1', 'β’', '2', 'β’', '3', 'β’', '4', 'β’', 'More']
['Gifts.', 'Users', 'can', 'now', 'send', 'gifts', 'to', 'each', 'other', 'β', 'celebrating', 'holidays', 'and', 'achievements', 'with', 'animated', 'artwork', 'and', 'custom', 'messages', '.', 'Gifts', 'are', 'purchased', 'with', 'Telegram', 'Stars', 'β', 'recipients', 'can', 'choose', 'to', 'display', 'gifts', 'on', 'their', 'profile', 'or', 'convert', 'them', 'back', 'to', 'Stars', 'for', 'their', 'own', 'balance.', 'October', 'Features', '1', 'β’', '2', 'β’', '3', 'β’', '4', 'β’', 'More']
['Due', 'to', 'the', 'recent', 'events', 'in', 'the', 'Middle', 'East,', 'particularly', 'in', 'Israel,', 'Lebanon,', 'and', 'Iran,', 'Telegram', 'experienced', 'unprecedented', 'loads.', 'In', 'the', 'past', 'few', 'days,', 'some', 'users', 'may', 'have', 'faced', 'temporary', 'connection', 'issues', 'and', 'difficulties', 'accessing', 'media.', 'Thanks', 'to', 'our', 'technical', "team's", 'efforts,', 'Telegram', 'remained', 'available', 'in', 'most', 'countries,', 'and', 'all', 'issues', 'are', 'now', 'fully', 'resolved.', 'We', 'apologize', 'for', 'any', 'inconvenience.']
['For', 'the', 'full', 'details', 'from', 'this', "week's", 'update,', 'including', '10', 'million', 'Premium', 'subscribers', 'and', 'dozens', 'of', 'bug', 'fixes,', 'check', 'out', 'our', 'latest', 'blog:', 'https://telegram.org/blog/star-giveaways-iv-in-browser', 'September', 'Features', '1', 'β’', '2', 'β’', '3', 'β’', 'More']
['Mini', 'App', 'Improvements.', 'Mini', 'apps', 'received', 'several', 'improvements,', 'making', 'them', 'smoother', 'and', 'more', 'responsive', '.', 'Developers', 'can', 'now', 'display', 'two', 'buttons', 'at', 'a', 'time', 'in', 'their', 'app,', 'in', 'multiple', 'orientations', 'or', 'with', 'a', 'shimmer', 'effect', '.', 'September', 'Features', '1', 'β’', '2', 'β’', '3', 'β’', 'More']
['Instant', 'View', 'for', 'Telegram', 'Browser.', 'Any', 'site', 'you', 'open', 'in', 'the', 'Telegram', 'Browser', 'can', 'be', 'converted', 'to', 'Instant', 'View', 'β', 'optimizing', 'articles', 'into', 'a', 'lightweight', 'layout', '.', 'September', 'Features', '1', 'β’', '2', 'β’', '3', 'β’', 'More']
['Star', 'Giveaways.', 'Giveaways', 'in', 'groups', 'and', 'channels', 'now', 'support', 'Telegram', 'Stars', 'β', 'which', 'winners', 'can', 'spend', 'on', 'paid', 'media,', 'subscriptions', 'and', 'mini', 'apps.', 'September', 'Features', '1', 'β’', '2', 'β’', '3', 'β’', 'More']
['π±', 'Telegram', 'abides', 'by', 'EU', 'laws,', 'including', 'the', 'Digital', 'Services', 'Act', 'β', 'its', 'moderation', 'is', 'within', 'industry', 'standards', 'and', 'constantly', 'improving.', 'π©', "Telegram's", 'CEO', 'Pavel', 'Durov', 'has', 'nothing', 'to', 'hide', 'and', 'travels', 'frequently', 'in', 'Europe.', 'π«€', 'It', 'is', 'absurd', 'to', 'claim', 'that', 'a', 'platform', 'or', 'its', 'owner', 'are', 'responsible', 'for', 'abuse', 'of', 'that', 'platform.', 'π', 'Almost', 'a', 'billion', 'users', 'globally', 'use', 'Telegram', 'as', 'means', 'of', 'communication', 'and', 'as', 'a', 'source', 'of', 'vital', 'information.', 'π', 'Weβre', 'awaiting', 'a', 'prompt', 'resolution', 'of', 'this', 'situation.', 'Telegram', 'is', 'with', 'you', 'all.']
['π', 'Telegram', 'celebrated', 'its', '11th', 'birthday', 'this', 'week', 'β', 'and', 'has', 'had', '17', 'major', 'updates', 'in', 'the', 'past', 'year.', 'For', 'the', 'full', 'details', '(and', 'features)', 'from', 'this', 'celebration,', 'check', 'out', 'our', 'blog:', 'https://telegram.org/blog/superchannels-star-reactions-subscriptions', 'August', 'Features', '1', 'β’', '2', 'β’', '3', 'β’', '4', 'β’', 'More']
['Super', 'Channels.', 'Channel', 'owners', 'can', 'make', 'their', 'feed', 'look', 'more', 'like', 'a', 'group', 'β', 'allowing', 'admins', 'to', 'post', 'as', 'their', 'own', 'profiles', 'or', 'other', 'channels', '.', 'August', 'Features', '1', 'β’', '2', 'β’', '3', 'β’', '4', 'β’', 'More']
['Paid', 'Media', 'for', 'Bots.', 'Bots', 'are', 'able', 'to', 'send', 'paid', 'media', 'β', 'to', 'support', 'their', 'services', 'and', 'automate', 'businesses', '.', 'August', 'Features', '1', 'β’', '2', 'β’', '3', 'β’', '4', 'β’', 'More']
['Star', 'Subscriptions', '.', 'Content', 'creators', 'can', 'make', 'special', 'invite', 'links', 'that', 'allow', 'users', 'to', 'join', 'a', 'channel', 'in', 'exchange', 'for', 'a', 'monthly', 'payment', 'in', 'Telegram', 'Stars', '.', 'August', 'Features', '1', 'β’', '2', 'β’', '3', 'β’', '4', 'β’', 'More']
['Star', 'Reactions.', 'Users', 'can', 'directly', 'support', 'their', 'favorite', 'channels', 'and', 'content', 'creators', 'by', 'sending', 'them', 'Telegram', 'Stars', 'with', 'a', 'new', 'βοΈ', 'Star', 'reaction', '.', 'August', 'Features', '1', 'β’', '2', 'β’', '3', 'β’', '4', 'β’', 'More']
['For', 'all', 'the', 'features', 'from', 'our', 'July', 'update', 'like', 'a', 'front', 'flash', 'for', 'video', 'messages', 'and', 'more', 'β', 'check', 'out', 'our', 'latest', 'blog:', 'https://telegram.org/blog/w3-browser-mini-app-store', 'July', 'Features', '1', 'β’', '2', 'β’', '3', 'β’', '4', 'β’', '5', 'β’', '6', 'β’', 'More']
['Story', 'Covers.', 'When', 'posting', 'a', 'video', 'story', 'to', 'your', 'profile,', 'you', 'can', 'choose', 'which', 'frame', 'to', 'use', 'as', 'its', 'cover', 'photo', '.', 'July', 'Features', '1', 'β’', '2', 'β’', '3', 'β’', '4', 'β’', '5', 'β’', '6', 'β’', 'More']
['Weather', 'Widget', 'in', 'Stories.', 'You', 'can', 'share', 'the', 'weather', 'in', 'your', 'stories', 'β', 'adding', 'an', 'animated', 'widget', 'that', 'automatically', 'converts', 'temperature', 'between', 'C', 'and', 'F', 'and', 'shows', 'moon', 'phases', 'at', 'night.', 'July', 'Features', '1', 'β’', '2', 'β’', '3', 'β’', '4', 'β’', '5', 'β’', '6', 'β’', 'More']
['Gifting', 'Telegram', 'Stars.', 'Telegram', 'Stars', 'can', 'be', 'purchased', 'for', 'your', 'contacts', 'β', 'giving', 'them', 'a', 'gift', 'to', 'spend', 'in', 'their', 'favorite', 'mini', 'apps', 'and', 'channels', '.', 'July', 'Features', '1', 'β’', '2', 'β’', '3', 'β’', '4', 'β’', '5', 'β’', '6', 'β’', 'More']
Epoch 1: 100%|ββββββββββ| 378/378 [00:01<00:00, 313.63it/s]
Epoch 2: 100%|ββββββββββ| 378/378 [00:01<00:00, 314.15it/s]
Epoch 3: 100%|ββββββββββ| 378/378 [00:01<00:00, 340.75it/s]
Epoch 4: 100%|ββββββββββ| 378/378 [00:01<00:00, 301.86it/s]
Epoch 5: 100%|ββββββββββ| 378/378 [00:01<00:00, 238.33it/s]
Epoch 6: 100%|ββββββββββ| 378/378 [00:01<00:00, 328.61it/s]
Epoch 7: 100%|ββββββββββ| 378/378 [00:01<00:00, 337.71it/s]
Epoch 8: 100%|ββββββββββ| 378/378 [00:01<00:00, 340.22it/s]
Epoch 9: 100%|ββββββββββ| 378/378 [00:01<00:00, 342.29it/s]
Epoch 10: 100%|ββββββββββ| 378/378 [00:01<00:00, 338.31it/s]
Epoch 11: 100%|ββββββββββ| 378/378 [00:01<00:00, 281.00it/s]
Epoch 12: 100%|ββββββββββ| 378/378 [00:01<00:00, 344.16it/s]
Epoch 13: 100%|ββββββββββ| 378/378 [00:01<00:00, 319.45it/s]
Epoch 14: 100%|ββββββββββ| 378/378 [00:01<00:00, 341.06it/s]
Epoch 15: 100%|ββββββββββ| 378/378 [00:01<00:00, 335.02it/s]
Epoch 16: 100%|ββββββββββ| 378/378 [00:01<00:00, 285.25it/s]
Epoch 17: 100%|ββββββββββ| 378/378 [00:01<00:00, 334.13it/s]
Epoch 18: 100%|ββββββββββ| 378/378 [00:01<00:00, 322.73it/s]
Epoch 19: 100%|ββββββββββ| 378/378 [00:01<00:00, 330.33it/s]
Epoch 20: 100%|ββββββββββ| 378/378 [00:01<00:00, 315.72it/s]
Epoch 21: 100%|ββββββββββ| 378/378 [00:01<00:00, 308.76it/s]
Epoch 22: 100%|ββββββββββ| 378/378 [00:01<00:00, 224.20it/s]
Epoch 23: 100%|ββββββββββ| 378/378 [00:01<00:00, 189.12it/s]
Epoch 24: 100%|ββββββββββ| 378/378 [00:02<00:00, 129.84it/s]
Epoch 25: 100%|ββββββββββ| 378/378 [00:02<00:00, 157.20it/s]
Epoch 26: 100%|ββββββββββ| 378/378 [00:02<00:00, 150.99it/s]
Epoch 27: 100%|ββββββββββ| 378/378 [00:01<00:00, 269.54it/s]
Epoch 28: 100%|ββββββββββ| 378/378 [00:01<00:00, 270.94it/s]
Epoch 29: 100%|ββββββββββ| 378/378 [00:01<00:00, 291.17it/s]
Epoch 30: 100%|ββββββββββ| 378/378 [00:01<00:00, 318.65it/s]
Epoch 31: 100%|ββββββββββ| 378/378 [00:01<00:00, 315.19it/s]
Epoch 32: 100%|ββββββββββ| 378/378 [00:01<00:00, 334.13it/s]
Epoch 33: 100%|ββββββββββ| 378/378 [00:01<00:00, 273.49it/s]
Epoch 34: 100%|ββββββββββ| 378/378 [00:01<00:00, 317.19it/s]
Epoch 35: 100%|ββββββββββ| 378/378 [00:01<00:00, 319.72it/s]
Epoch 36: 100%|ββββββββββ| 378/378 [00:01<00:00, 335.90it/s]
Epoch 37: 100%|ββββββββββ| 378/378 [00:01<00:00, 321.90it/s]
Epoch 38: 100%|ββββββββββ| 378/378 [00:01<00:00, 314.41it/s]
Epoch 39: 100%|ββββββββββ| 378/378 [00:01<00:00, 213.93it/s]
Epoch 40: 100%|ββββββββββ| 378/378 [00:01<00:00, 203.79it/s]
Epoch 41: 100%|ββββββββββ| 378/378 [00:01<00:00, 230.35it/s]
Epoch 42: 100%|ββββββββββ| 378/378 [00:01<00:00, 204.78it/s]
Epoch 43: 100%|ββββββββββ| 378/378 [00:01<00:00, 250.98it/s]
Epoch 44: 100%|ββββββββββ| 378/378 [00:01<00:00, 291.40it/s]
Epoch 45: 100%|ββββββββββ| 378/378 [00:01<00:00, 319.18it/s]
Epoch 46: 100%|ββββββββββ| 378/378 [00:01<00:00, 265.43it/s]
Epoch 47: 100%|ββββββββββ| 378/378 [00:01<00:00, 329.76it/s]
Epoch 48: 100%|ββββββββββ| 378/378 [00:01<00:00, 303.32it/s]
Epoch 49: 100%|ββββββββββ| 378/378 [00:01<00:00, 255.22it/s]
Epoch 50: 100%|ββββββββββ| 378/378 [00:01<00:00, 261.57it/s]
Epoch 51: 100%|ββββββββββ| 378/378 [00:01<00:00, 272.70it/s]
Epoch 52: 100%|ββββββββββ| 378/378 [00:01<00:00, 248.19it/s]
Epoch 53: 100%|ββββββββββ| 378/378 [00:01<00:00, 332.95it/s]
Epoch 54: 100%|ββββββββββ| 378/378 [00:01<00:00, 315.46it/s]
Epoch 55: 100%|ββββββββββ| 378/378 [00:01<00:00, 279.76it/s]
Epoch 56: 100%|ββββββββββ| 378/378 [00:02<00:00, 174.70it/s]
Epoch 57: 100%|ββββββββββ| 378/378 [00:01<00:00, 326.63it/s]
Epoch 58: 100%|ββββββββββ| 378/378 [00:01<00:00, 343.54it/s]
Epoch 59: 100%|ββββββββββ| 378/378 [00:01<00:00, 199.70it/s]
Epoch 60: 100%|ββββββββββ| 378/378 [00:01<00:00, 221.06it/s]
Epoch 61: 100%|ββββββββββ| 378/378 [00:01<00:00, 317.31it/s]
Epoch 62: 100%|ββββββββββ| 378/378 [00:01<00:00, 264.31it/s]
Epoch 63: 100%|ββββββββββ| 378/378 [00:01<00:00, 262.30it/s]
Epoch 64: 100%|ββββββββββ| 378/378 [00:01<00:00, 313.63it/s]
Epoch 65: 100%|ββββββββββ| 378/378 [00:01<00:00, 317.58it/s]
Epoch 66: 100%|ββββββββββ| 378/378 [00:01<00:00, 297.35it/s]
Epoch 67: 100%|ββββββββββ| 378/378 [00:01<00:00, 239.24it/s]
Epoch 68: 100%|ββββββββββ| 378/378 [00:01<00:00, 314.15it/s]
Epoch 69: 100%|ββββββββββ| 378/378 [00:01<00:00, 242.92it/s]
Epoch 70: 100%|ββββββββββ| 378/378 [00:01<00:00, 319.18it/s]
Epoch 71: 100%|ββββββββββ| 378/378 [00:01<00:00, 329.47it/s]
Epoch 72: 100%|ββββββββββ| 378/378 [00:01<00:00, 317.31it/s]
Epoch 73: 100%|ββββββββββ| 378/378 [00:01<00:00, 335.91it/s]
Epoch 74: 100%|ββββββββββ| 378/378 [00:01<00:00, 284.60it/s]
Epoch 75: 100%|ββββββββββ| 378/378 [00:01<00:00, 317.84it/s]
Epoch 76: 100%|ββββββββββ| 378/378 [00:01<00:00, 323.28it/s]
Epoch 77: 100%|ββββββββββ| 378/378 [00:01<00:00, 317.58it/s]
Epoch 78: 100%|ββββββββββ| 378/378 [00:01<00:00, 317.31it/s]
Epoch 79: 100%|ββββββββββ| 378/378 [00:01<00:00, 267.68it/s]
Epoch 80: 100%|ββββββββββ| 378/378 [00:01<00:00, 337.71it/s]
Epoch 81: 100%|ββββββββββ| 378/378 [00:01<00:00, 322.72it/s]
Epoch 82: 100%|ββββββββββ| 378/378 [00:01<00:00, 314.15it/s]
Epoch 83: 100%|ββββββββββ| 378/378 [00:01<00:00, 338.92it/s]
Epoch 84: 100%|ββββββββββ| 378/378 [00:01<00:00, 265.43it/s]
Epoch 85: 100%|ββββββββββ| 378/378 [00:01<00:00, 328.04it/s]
Epoch 86: 100%|ββββββββββ| 378/378 [00:01<00:00, 329.18it/s]
Epoch 87: 100%|ββββββββββ| 378/378 [00:01<00:00, 330.62it/s]
Epoch 88: 100%|ββββββββββ| 378/378 [00:01<00:00, 338.91it/s]
Epoch 89: 100%|ββββββββββ| 378/378 [00:01<00:00, 250.15it/s]
Epoch 90: 100%|ββββββββββ| 378/378 [00:01<00:00, 300.66it/s]
Epoch 91: 100%|ββββββββββ| 378/378 [00:01<00:00, 289.83it/s]
Epoch 92: 100%|ββββββββββ| 378/378 [00:01<00:00, 321.35it/s]
Epoch 93: 100%|ββββββββββ| 378/378 [00:01<00:00, 321.08it/s]
Epoch 94: 100%|ββββββββββ| 378/378 [00:01<00:00, 256.60it/s]
Epoch 95: 100%|ββββββββββ| 378/378 [00:01<00:00, 309.26it/s]
Epoch 96: 100%|ββββββββββ| 378/378 [00:01<00:00, 332.95it/s]
Epoch 97: 100%|ββββββββββ| 378/378 [00:01<00:00, 333.54it/s]
Epoch 98: 100%|ββββββββββ| 378/378 [00:01<00:00, 295.04it/s]
Epoch 99: 100%|ββββββββββ| 378/378 [00:01<00:00, 314.41it/s]
Epoch 100: 100%|ββββββββββ| 378/378 [00:01<00:00, 334.72it/s]
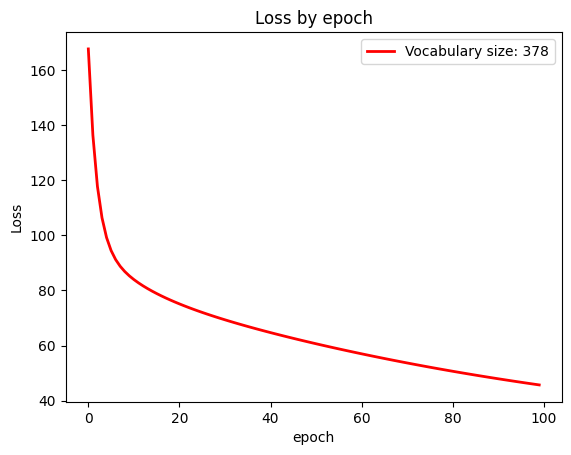
Vector of "'Telegram'" word:
[ 1.55561541e-01 1.77020706e-01 -5.40811034e-03 3.59769057e-03
-8.16132010e-03 -4.15956336e-02 -1.79394031e-02 -1.44513644e-01
1.56769822e-01 -5.83205062e-05 1.55230251e-01 1.15323020e-02
1.20502608e-01 -2.04556712e-01 -1.29421676e-01 3.21621670e-02
-1.63679719e-01 -1.14871509e-01 8.62045380e-02 -5.56635456e-02
-1.67371505e-01 -5.28203969e-02 -9.17762628e-02 1.08312141e-01
-5.51253747e-02 1.67345727e-01 1.61171293e-01 -6.27636788e-03
1.39057885e-01 1.83056904e-01 2.28870038e-01 -1.28071171e-01
-1.26117661e-01 4.29829807e-02 -2.03594756e-01 -1.60113889e-01
-2.32977882e-02 1.37207303e-02 -2.25264920e-01 2.30003619e-01
1.33797845e-01 -6.34702484e-02 -1.32517611e-01 5.88101981e-02
2.36643770e-01 1.24257856e-01 -3.05104777e-01 -2.45094159e-02
3.45571410e-02 -4.56085027e-02], word_id:176
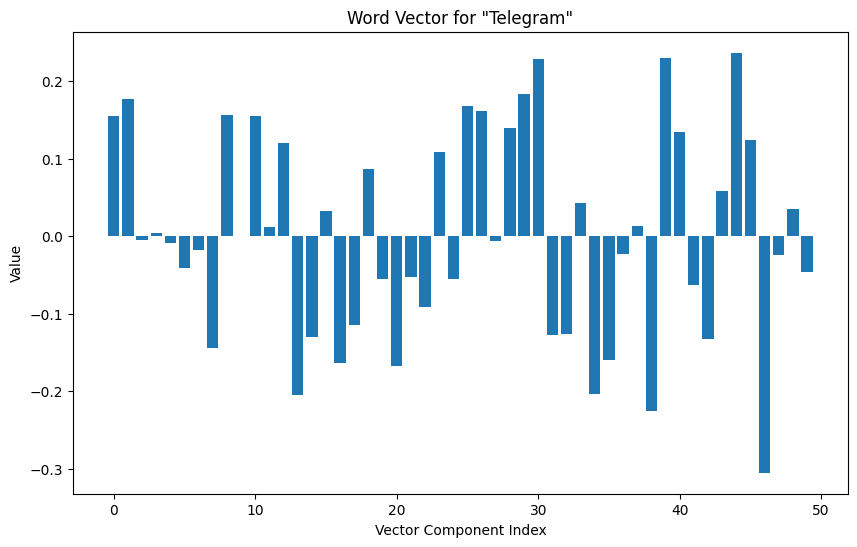
Why GloVe is Effective ?#
Global Information: GloVe leverages the global co-occurrence statistics of the entire corpus, meaning it takes into account all the word pairs and their relationships across the whole text.
Semantic Vectors: The resulting word embeddings capture semantic relationships, meaning that words like βkingβ and βqueenβ will have similar vector representations because of their similar usage contexts.
Linear Relationships: One of GloVeβs key features is its ability to represent linear relationships between words. For example, it captures analogies like: vector(βkingβ) - vector(βmanβ) + vector(βwomanβ) β vector(βqueenβ) This means that GloVe can model relationships between words in a way that reflects their real-world meanings.
Efficient Representation: Words with similar meanings or usage patterns will have similar vectors, which is useful for downstream NLP tasks.
Applications for GloVe:#
Text Analysis: Used in NLP tasks to capture word meanings and relationships for tasks like sentiment analysis or text classification.
Recommendation Systems: Helps find relationships between words or items for smarter recommendations.
Machine Translation: Improves machine translation systems by providing better word representations based on their meaning.
Difference Between GloVe and Word2Vec:#
GloVe focuses on the global co-occurrence of words in a corpus and uses a matrix to capture these relationships. Word2Vec, on the other hand, is based on local context windows around words and predicts word occurrences based on nearby words.
GloVe generally excels at learning more general semantic relationships between words, whereas Word2Vec focuses more on predictive word modeling.
Conclusion:#
GloVe is a powerful word embedding model that learns to represent words as vectors by analyzing how often they co-occur with other words in a corpus. These embeddings capture both the meaning and relationships between words, making GloVe extremely useful in various NLP tasks. Its ability to encode semantic relationships, including analogies, makes it one of the leading methods for generating word embeddings, alongside Word2Vec.
Refrences:#
3: An Introduction to the Global Vectors (GloVe) Algorithm
4: GloVe in NLP